|
Cityscapes
数据集官网:Semantic Understanding of Urban Street Scenes
对应论文:The cityscapes dataset for semantic urban scene understanding (2016CVPR)
单位:Daimler AG R&D戴姆勒集团,达姆施塔特工业大学等
作者:Marius Cordts,Mohamed Omran,Sebastian Ramos等
数据集简介:含有5000张精标图像以及20000张粗标图像。5000张精标图中训练集、验证集和测试集分别包含2975,500,1525图像。场景上:季节上有春、夏和秋天;50个城市,主要在德国(以及相邻国家);主要是良好的天气,不包含如大雨和大雾的恶劣天气。分割任务中评估类别共19类:road, sidewalk, building, wall, fence, pole, traffic light, traffic sign, vegetation, terrain, sky, person, rider, car, truck, bus, train, motorcycle, bicycle。
任务:语义分割、实例分割、全景分割、3D车辆检测
SYNTHIA-RAND-Cityscapes
数据集官网:The SYNTHIA dataset
对应论文:The synthia dataset: A large collection of synthetic images for semantic segmentation of urban scenes (2016CVPR)
单位:巴塞罗那自治大学,维也纳大学
作者:German Ros,Laura Sellart,Joanna Materzynska,等
数据集简介:自动驾驶仿真数据集。9,000张带标注图像。类别定义与Cityscapes一致,包含:void, sky, building, road, sidewalk, fence, vegetation, pole, car, traffic sign, pedestrian, bicycle, motorcycle, parking-slot, road-work, traffic light, terrain, rider, truck, bus, train, wall, lanemarking。一般在实验中使用16个类别。包含四季、光照、相机角度变化。本文想要探究的是合成图像对语义分割任务有多大的帮助,还有对应的视频数据集成为SYNTHIA-Seqs
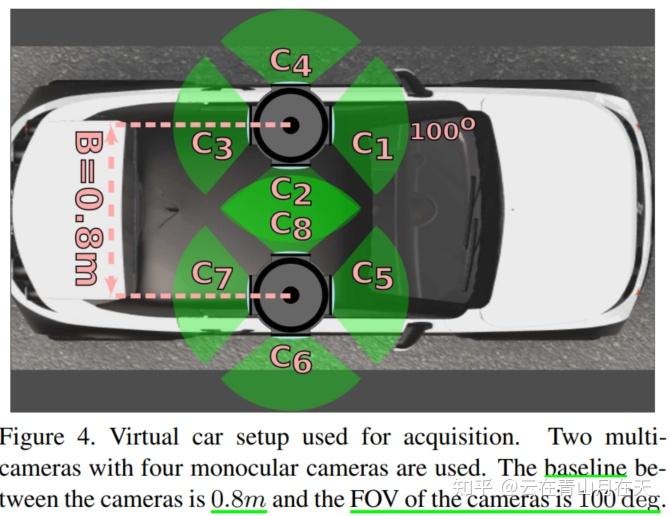
视频数据捕捉用的传感器设置[1]
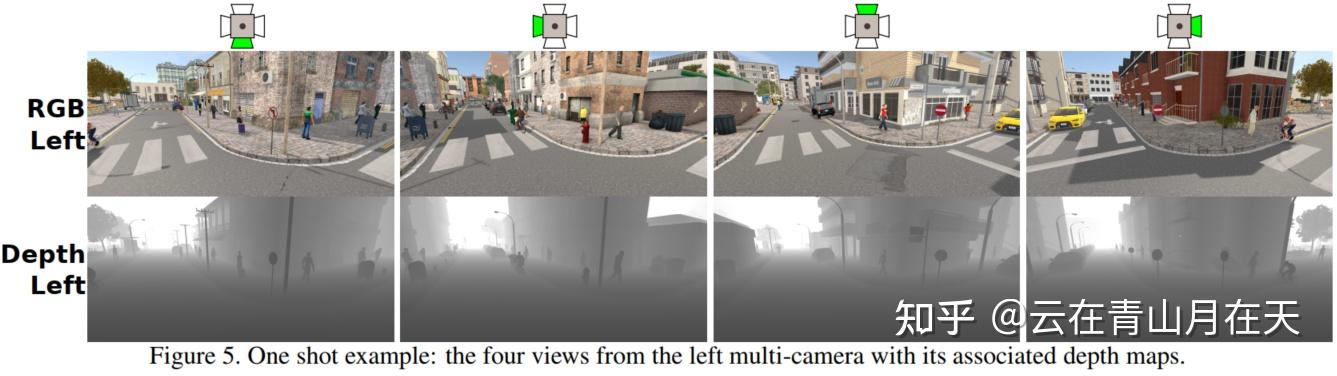
四个方向相机捕捉的图像展示[1]
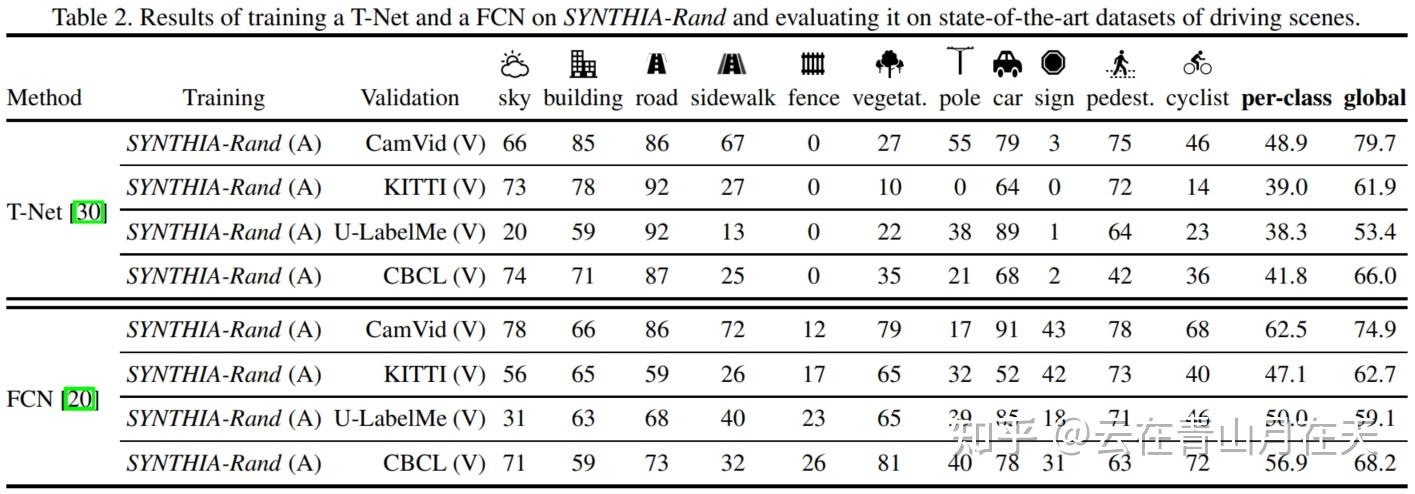
只使用仿真数据集训练语义分割模型的结果[1]
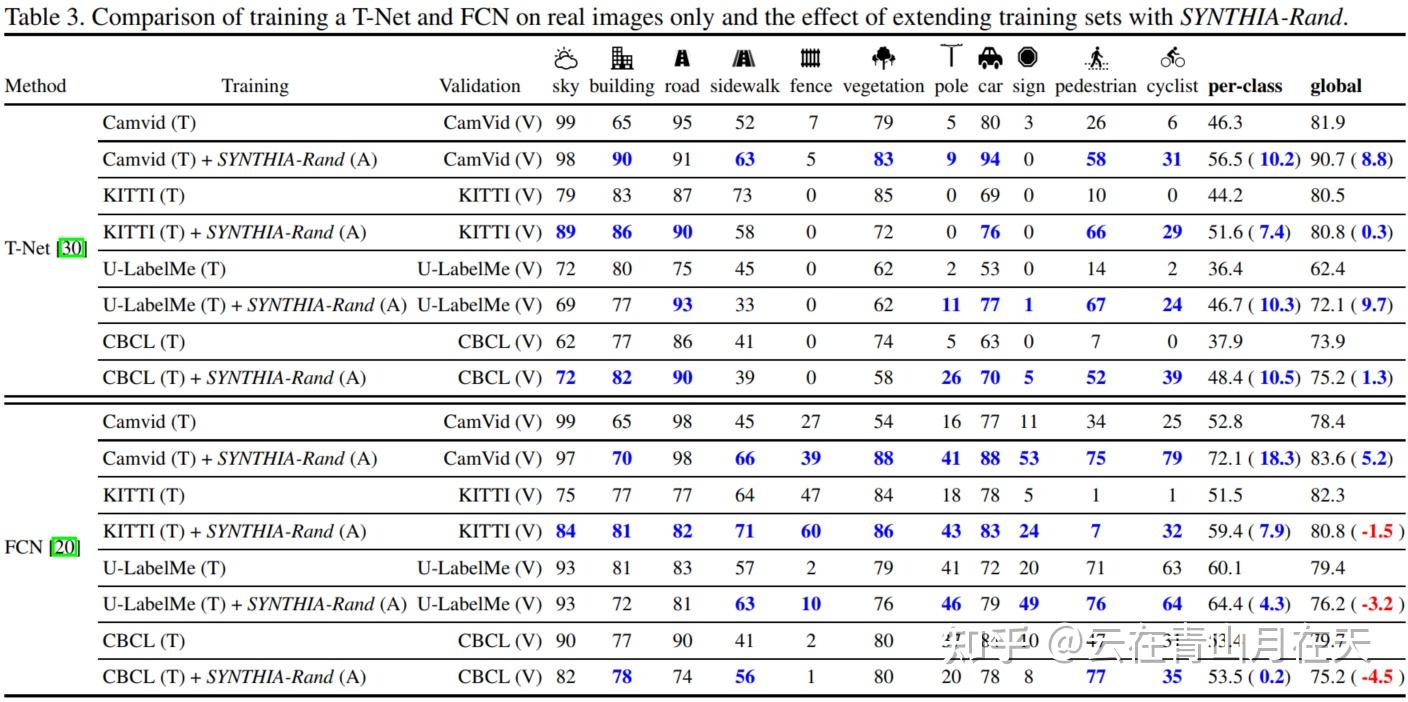
只使用真实数据集、同时使用仿真和真实数据集训练语义分割模型的结果[1]
任务:语义分割、实例分割、深度估计、无监督域适应(从仿真到真实,SYNTHIA->Cityscapes)
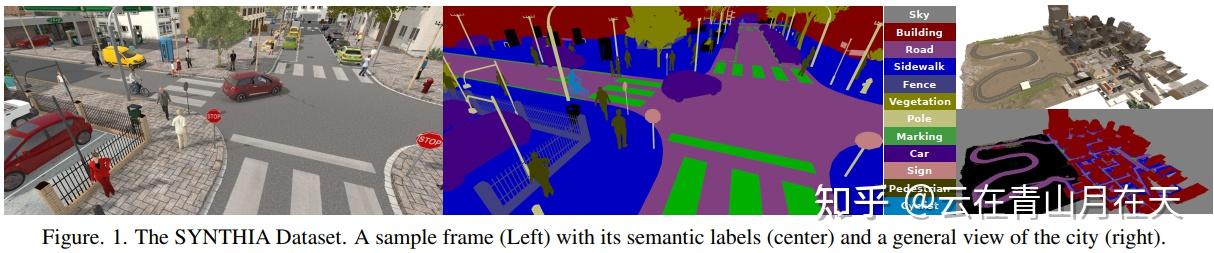
仿真数据集展示[1]
GTA5
数据集官网:Playing for Data: Ground Truth from Computer Games
对应论文:Playing for data: Ground truth from computer games (ECCV2017)
单位:达姆施塔特工业大学,因特尔实验室
作者:Stephan R. Richter,Vibhav Vineet, Stefan Roth, and Vladlen Koltun
数据集简介:自动驾驶仿真数据集。24966张带标注图像。类别定义与Cityscapes一致,包含:road, building, sky, sidewalk, vegetation, cat, terrain, wall, truck, poole, fence, bus, person, traffic light, traffic sign, traink motorcycle, rider, bicycle。一般在实验中使用19个类别。
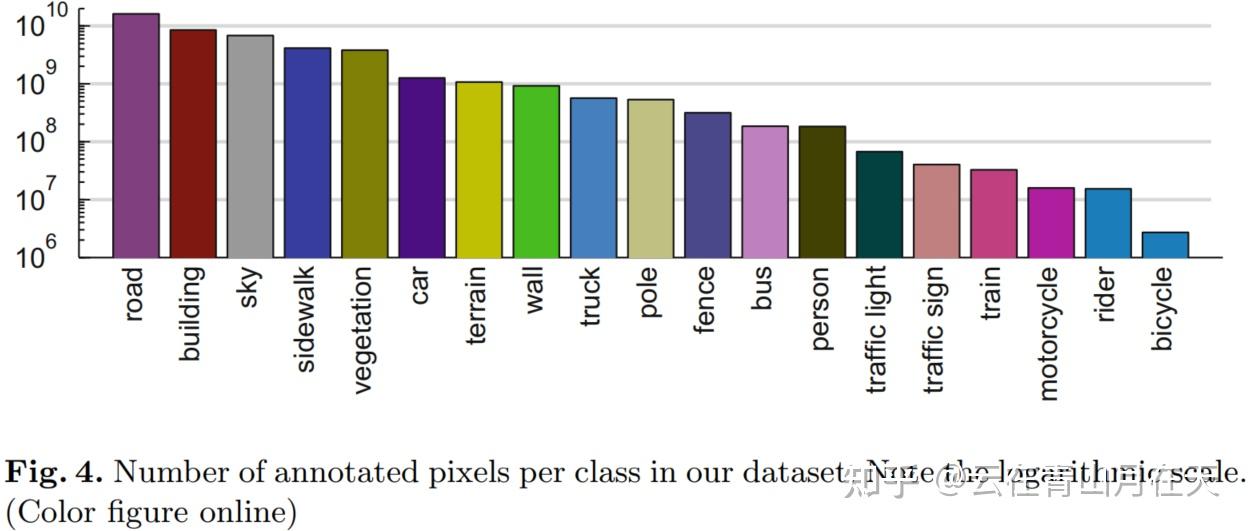
各类别物体像素分布[2]
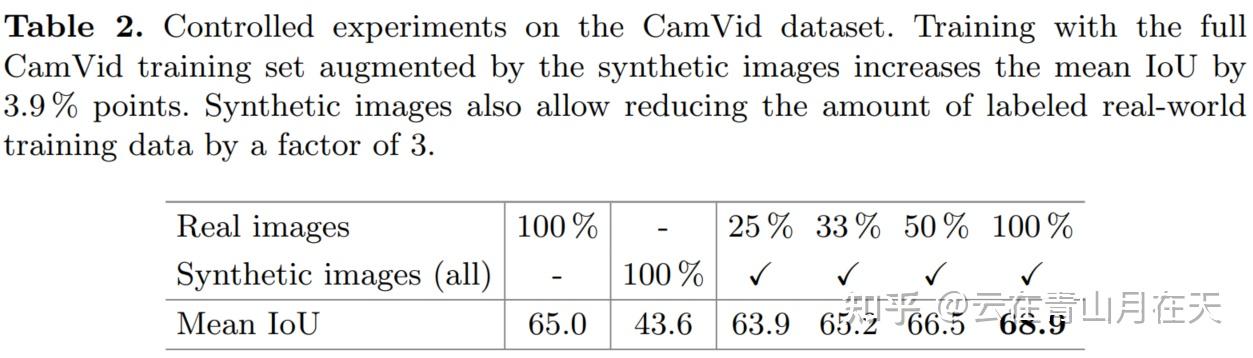
使用GTA5数据集辅助CamVid训练语义分割模型,仅使用1/3的真实数据就可以达到完整真实数据集的训练效果[2]
任务:语义分割
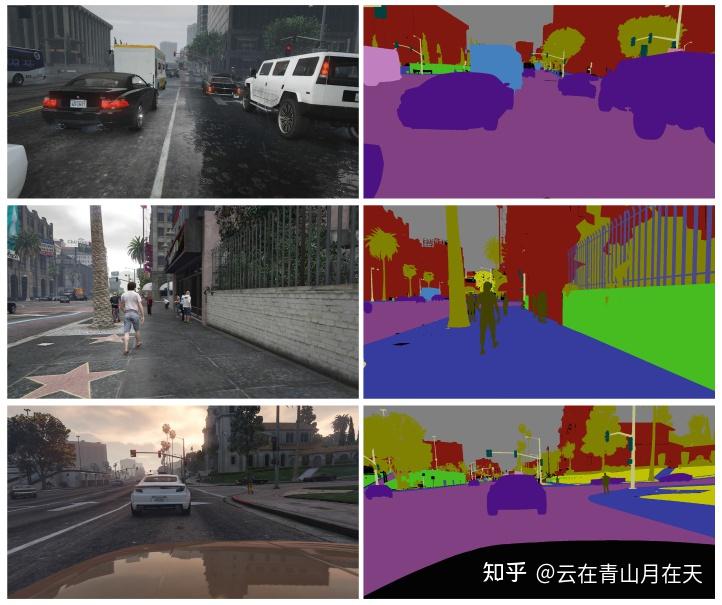
仿真数据集展示
VIPER
数据集官网:Playing for Benchmarks 含有语义、实例、全景分割,深度估计,视觉里程计,光流估计
对应论文:Playing for benchmarks (ICCV2017)
github地址: https://github.com/srrichter/viper
单位:达姆施塔特工业大学等
作者:Stephan R. Richter,Zeeshan Hayder,Vladlen Koltun
数据集简介:自动驾驶仿真数据集,由GTA5产生,分辨率为1920*1080。总共有254064帧标注图像,训练、验证和测试集包含的图像数量分别为134k,50k和70k。包含白天、日落、下雨、下雪和夜间场景。标注了像素级语义类别、实例级语义、实例级语义边界、物体检测和跟踪、3D物体检测信息(位置和朝向)、光流和自我运动(ego motion)
任务(提供baseline):语义分割、实例分割、光流估计、视觉里程计
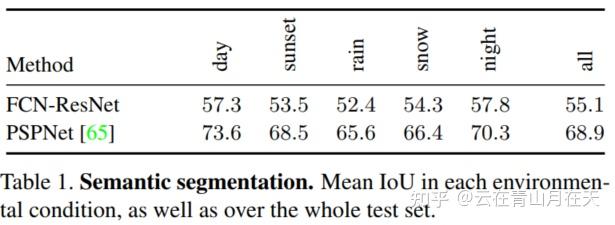
语义分割baseline[3]
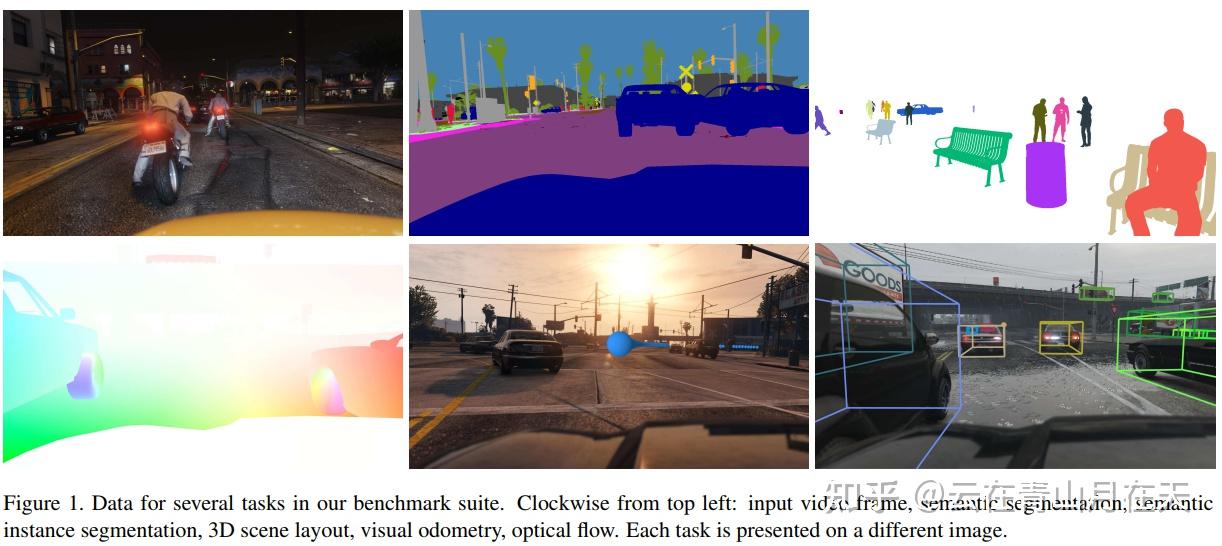
仿真数据集展示[3]
Maipllary Vistas
数据集官网:https://www.mapillary.com/dataset/vistas
对应论文:ICCV 2017 Open Access Repository (ICCV2017)
单位:research@mapillary.com
作者:Gerhard Neuhold, Tobias Ollmann, Samuel Rota Bulo等
数据集简介:含有25000张精标图像,训练、验证和测试集分别包含18000,2000和5000张图像。收集地点包括欧洲、美洲、亚洲、非洲、大洋洲,包括光照、天气、季节、地理环境的多样性。包含66个语义类别以及37个实例类别。
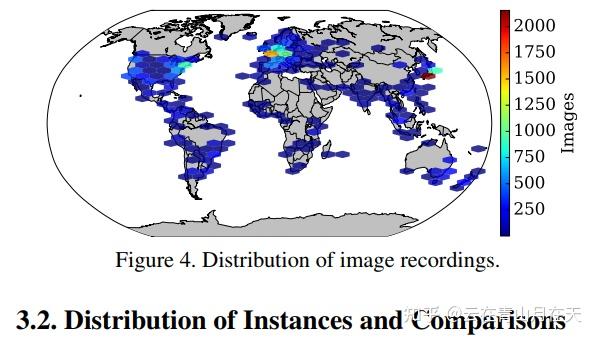
Mapillary Vistas收集地理位置[4]
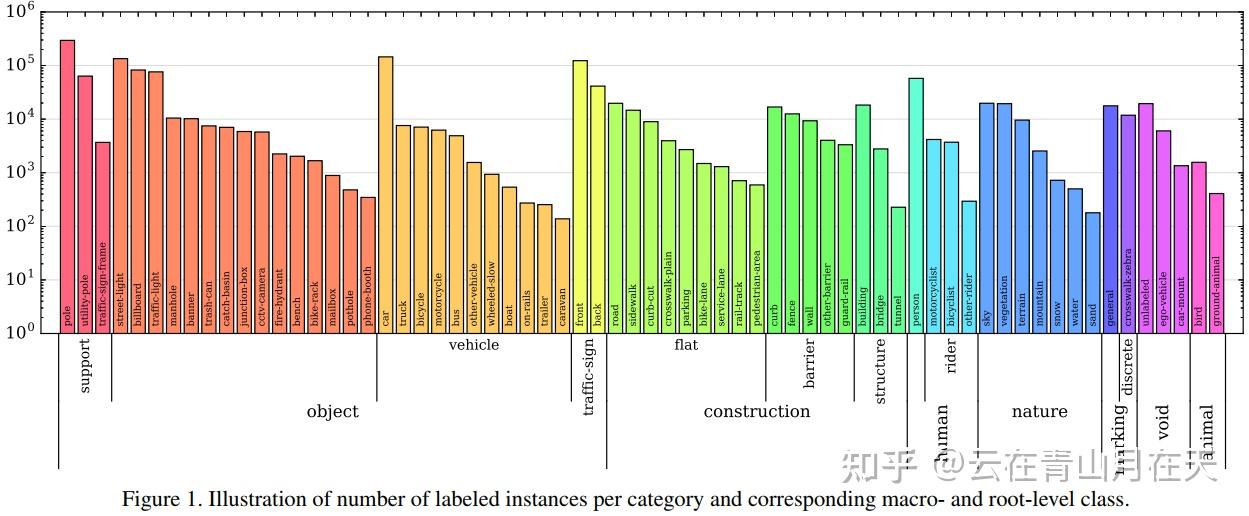
Mapillary Vistas中的类别分布[4]
任务:语义分割、实例分割、全景分割
Foggy Cityscapes/Foggy Driving
数据集官网:Semantic Foggy Scene Understanding with Synthetic Data
对应论文:Semantic foggy scene understanding with synthetic data (IJCV2018)
单位:苏黎世联邦理工学院、鲁汶大学
作者:Christos Sakaridis,Dengxin Dai,Luc Van Gool
数据集简介:Foggy Cityscapes是将雾合成到真实的天气良好的Cityscapes数据集上得到的,用于辅助雾天分割/检测任务,20000张Foggy Cityscapes-coarse,550张精细化的Foggy Cityscapes-refined(498用于训练,52用于验证),类别与Cityscapes保持一致;Foggy Driving是真实采集和标注的雾天数据集,101张,其中51张来自手机拍摄,50张从网上搜集,分辨率960*1280,类别与Cityscapes保持一致,33张精标,68张粗标,该数据集主要用于测试。
任务:语义分割、物体检测

雾天图像展示(不同可见度)[5]
Foggy Zurich
数据集官网:Model Adaptation with Synthetic and Real Data for Semantic Dense Foggy Scene Understanding
对应论文:Model adaptation with synthetic and real data for semantic dense foggy scene understanding(ECCV2018)
单位:苏黎世联邦理工学院、鲁汶大学
作者:Christos Sakaridis, Dengxin Dai, Simon Hecker, and Luc Van Gool
数据集简介:Foggy Zurich真实的雾天场景数据集,总共采集了3808张图像。只选择了16张进行标注,分辨率1920*1080,类别与Cityscapes保持一致19类,该数据集主要用于测试。
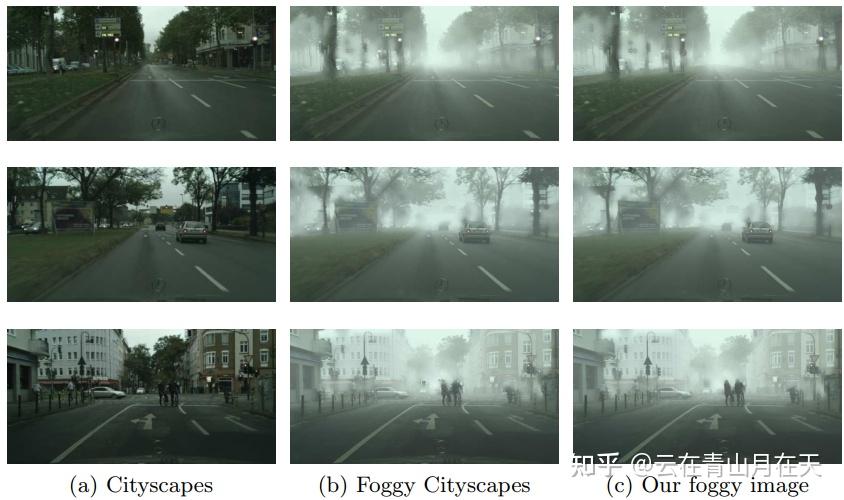
雾天图像效果展示[6]
Weather Kitti and Weather Cityscapes
数据集官网:Physics-Based Rendering for Improving Robustness to Rain
对应论文:Physics-based rendering for improving robustness to rain (ICCV2019)
单位:法国国家信息与自动化研究所,拉瓦尔大学
作者:Shirsendu Sukanta Halder等
代码:GitHub - cv-rits/rain-rendering: Rain Rendering for Evaluating and Improving Robustness to Bad Weather (Tremblay et al., 2020) (S. S. Halder et al., 2019)
数据集简介:有不同雨量的增强KITTI和Cityscapes数据集。类别与原数据集保持一致。
任务:语义分割、物体检测
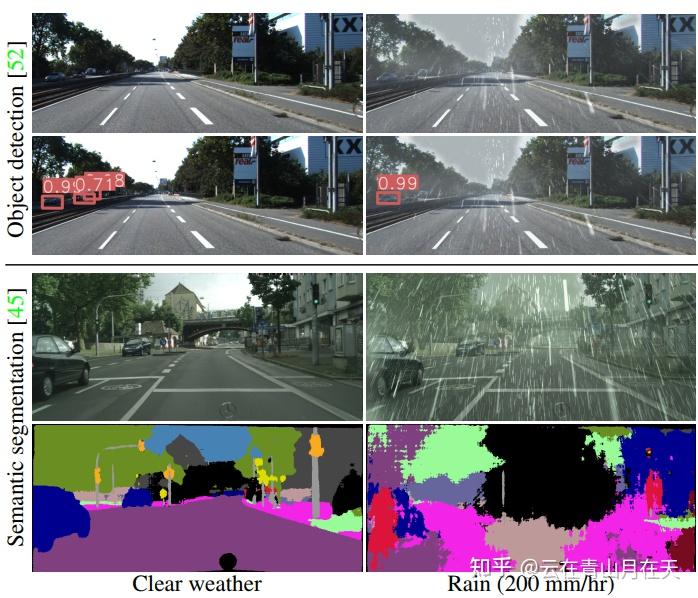
良好天气和雨天下物体检测和语义分割效果对比[7]
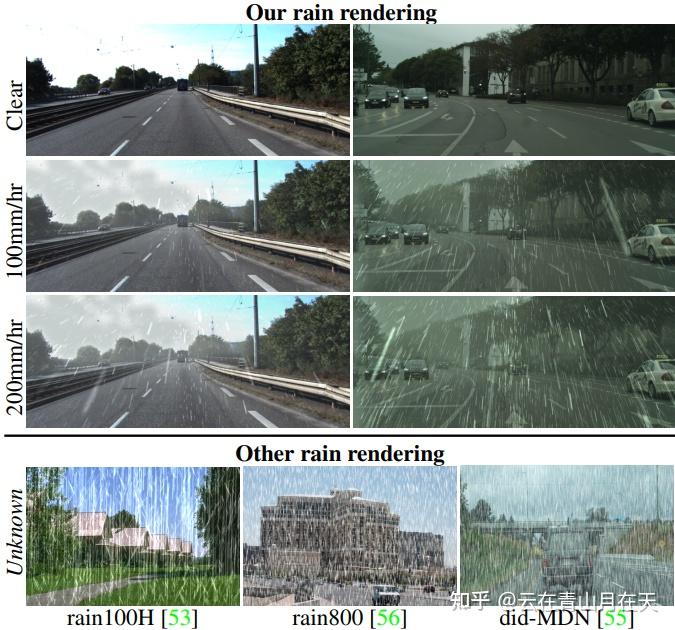
雨天的合成效果展示以及对比[7]
BDD100K
数据集官网:BDD100K
对应论文:Bdd100k: A diverse driving dataset for heterogeneous multitask learning (2020CVPR)
单位:UC伯克利,康奈尔大学,UCSD等
作者:Fisher Yu, Haofeng Chen, Xin Wang等
数据集简介:BDD100K是驾驶场景视频数据集,包含100K个视频序列,10种任务。在地理、环境以及天气上具有多样性。在美国搜集的。
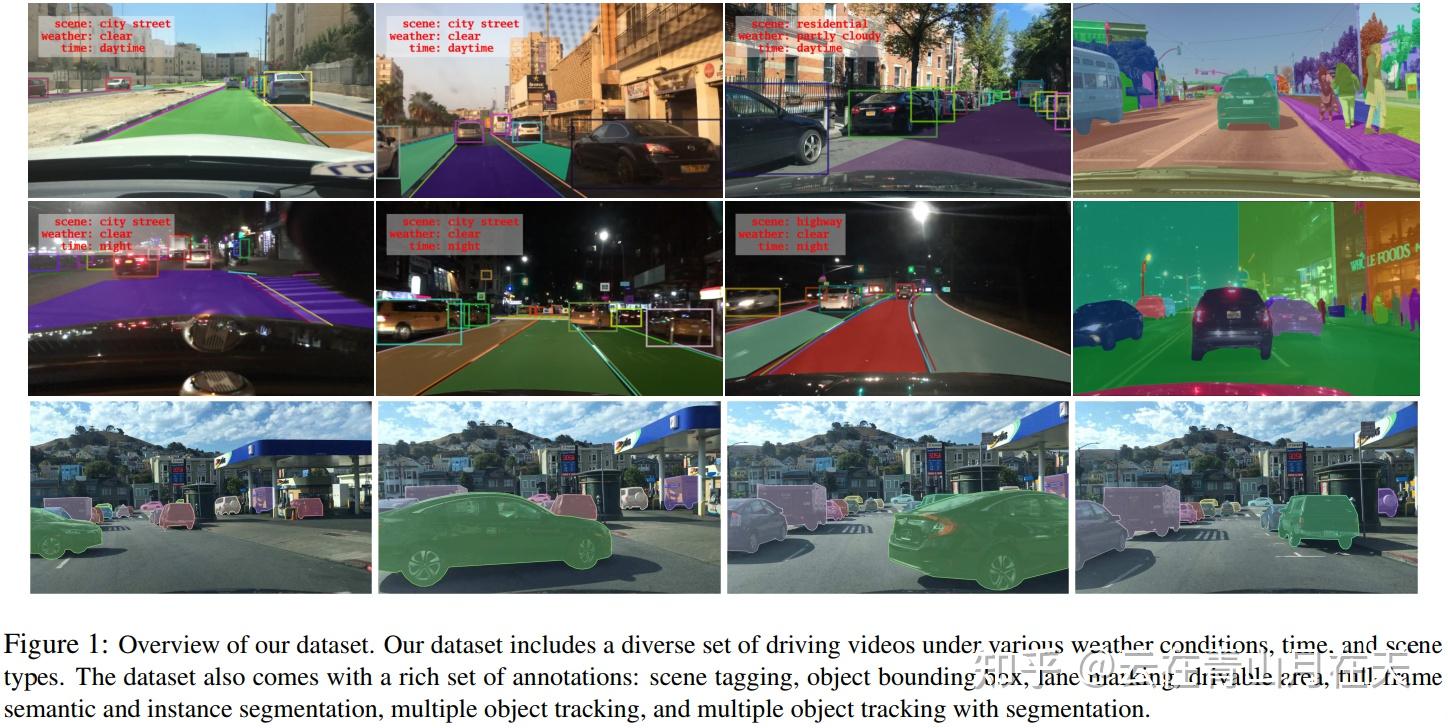
BDD100k[8]
任务:物体检测、实例分割、语义分割、多物体跟踪、多物体跟踪与分割、车道线检测、可行驶区域检测、图像标记(Image Tagging)、模仿学习(Imitation Learning)、域适应(Domain Adaptation)
ApolloScape
数据集官网:Apollo Scape
对应论文:The apolloscape open dataset for autonomous driving and its application (2020PAMI)
单位:百度
作者:Xinyu Huang,Peng Wang,Xinjing Cheng等
数据集简介:主要在中国搜集的自动驾驶数据集。包含了密集的3D点云标注、立体驾驶视频、高精度的6自由度相机位姿、不同时间下的视频(早中晚)、密集的帧级语义标签(35类别、144K+ imgs)、像素级的路标标注(35类别,160K+ imgs),2D实例分割(8类别,90K+imgs)、2D车关键点以及3D车实例标注(70K+车)
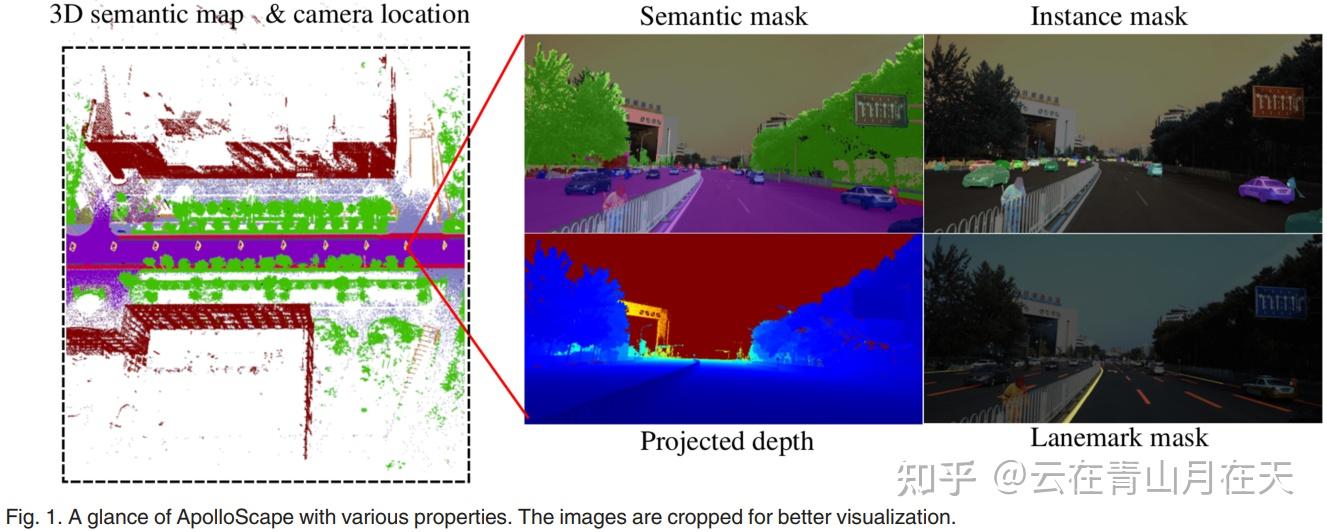
ApolloScape[9]
任务:Scene Parsing, Car Instance, Lane Segmentation, Localization, Trajectory, Detection/Tracking, Stereo
ACDC
数据集官网:https://acdc.vision.ee.ethz.ch
对应论文:ACDC: The adverse conditions dataset with correspondences for semantic driving scene understanding (ICCV2021)
单位:ETH等
作者:Christos Sakaridis, Dengxin Dai, and Luc Van Gool
数据集简介:ACDC数据集包含了4006张图像,它们均匀地分布在四种常见的不利天气条件下:雾天,夜间,雨天和雪天。每张不利条件的图像有高质量的像素级标注以及对应的在正常条件下几乎相同场景采集的图像,以及一个二值掩膜来区分图内清晰和不确定的语义内容。因此,ACDC数据集能够支持标准的语义分割以及新提出的具有不确定意识的语义分割任务。雾、黑夜、雨、雪天气分别采集了1000,1006,1000,1000张图像,每种条件的训练、验证和测试集分为400, 100(106)和500,总的划分为1600,406,2000。

ACDC标注过程[1]
任务:(不利天气下的)语义分割、具有不确定性意识的语义分割
云在青山月在天:ACDC数据集论文阅读与使用测试
Ithaca365
数据集官网:A new dataset to enable robust autonomous driving via a novel data collection process
论文:Ithaca365: Dataset and Driving Perception Under Repeated and Challenging Weather Conditions (CVPR2022)
单位:康奈尔大学等
作者:Carlos A. Diaz-Ruiz等
数据集简介:本文提出了一个实现鲁棒自主驾驶的数据集,采用一个新数据收集过程,即在不同场景(城市、公路、农村、校园)、天气(雪、雨、太阳)、时间(白天/晚上)和交通条件(行人、自行车手和汽车)下,沿着15公里的路线重复记录数据。该数据集包括来自摄像机和激光雷达传感器的图像和点云,以及高精度GPS/INS,建立跨路线的对应关系。该数据集包括道路和目标标注,具有非模态(amodal)掩码捕捉的局部遮挡和3-D边框。重复路径为目标发现、连续学习和异常检测开辟了新的研究方向。https://zhuanlan.zhihu.com/p/549714042 任务:Amodal道路分割、Amodal实例分割、3D物体检测,立体视差估计
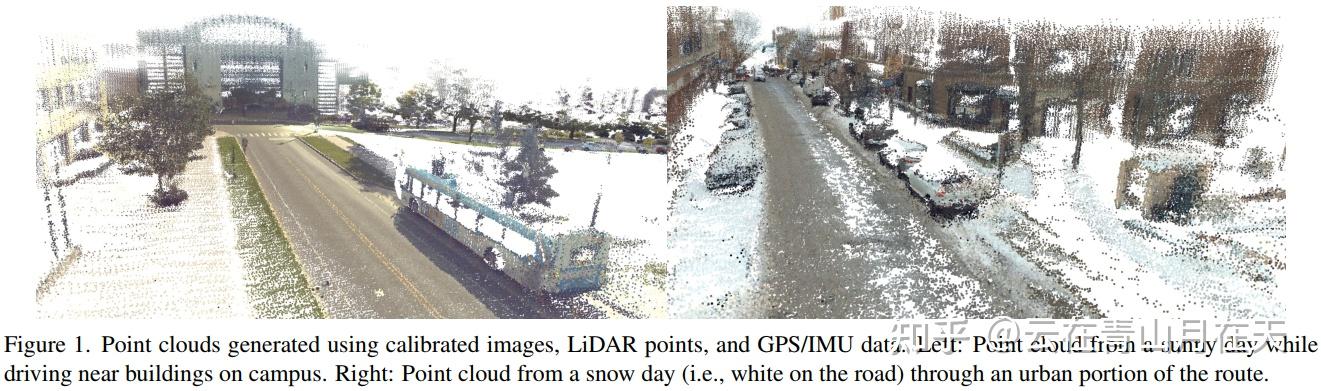
标定后利用图像、点云、GPS/IMU数据生成的点云图[11]
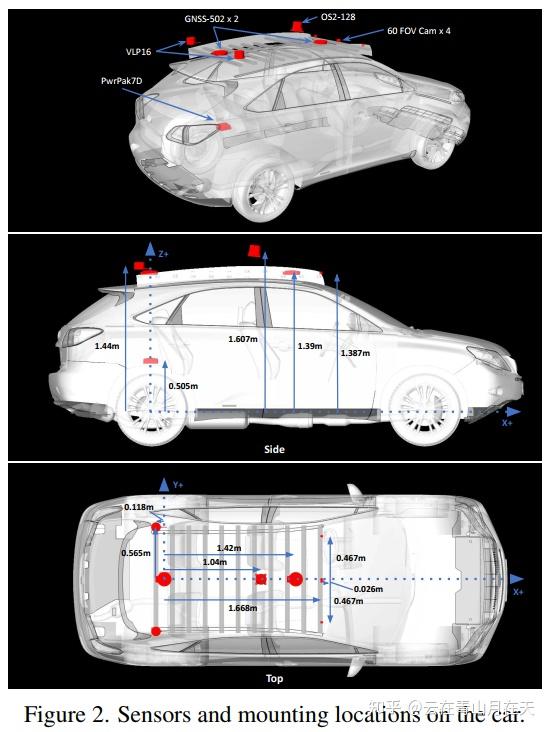
传感器安装示意图[11]
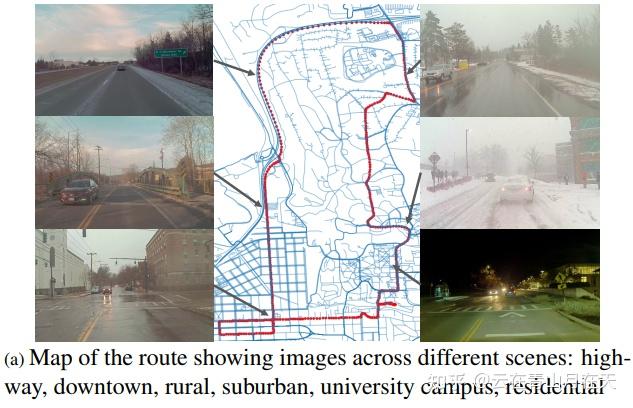
15km道路上不同位置的场景图[11]
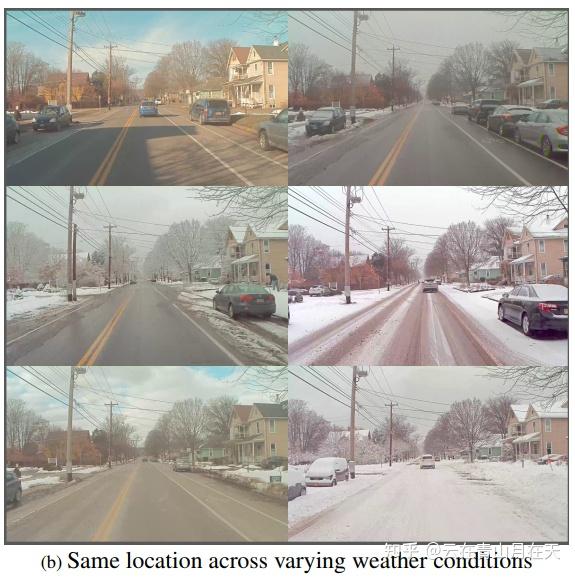
相同位置不同环境条件的示意图[11]
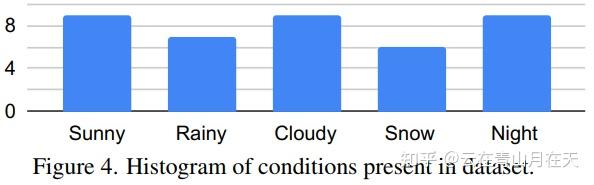
数据集中各个条件的比例[11]

不同天气条件下汽车的 amodal 地面实况掩码示例[11]
SHIFT
数据集官网:SHIFT Dataset
论文地址:SHIFT: A Synthetic Driving Dataset for Continuous Multi-Task Domain Adaptation (CVPR2022)
单位:KTH等
作者:Tao Sun, Mattia Segu, Janis Postels等
数据集简介:本文提出了一个包含离散和连续域偏移的多任务驾驶场景仿真数据集SIFT,包含了多样任务的标注并且包含了多种驾驶条件。使用CARLA仿真器搜集的数据集
任务:语义/实例分割、单目/立体深度回归、2D/3D 对象检测、2D/3D 多重目标跟踪 (MOT)、光流估计、点云配准、视觉里程计、轨迹预测和人体姿态估计。
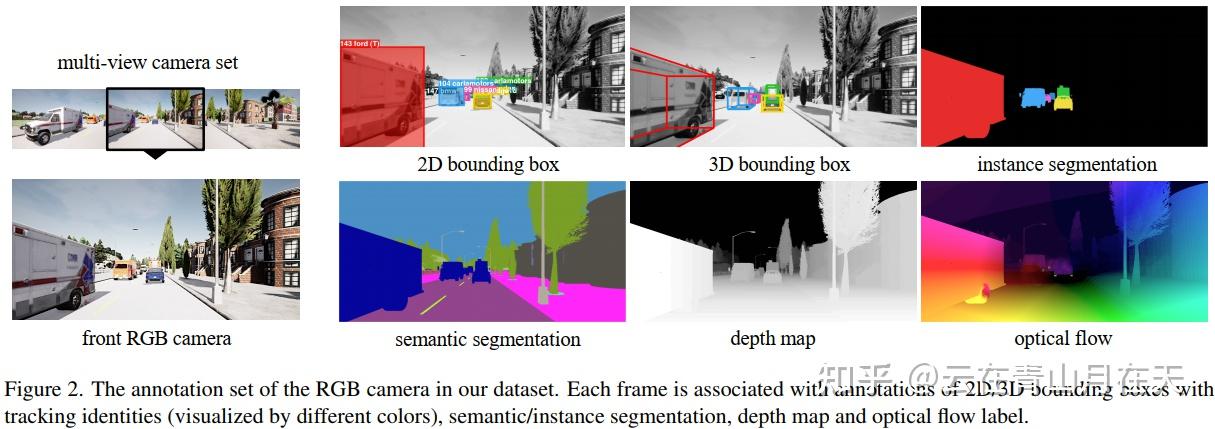
云在青山月在天:SHIFT: A Synthetic Driving Dataset for Continuous Multi-Task Domain Adaptation论文阅读笔记
[1] Ros G, Sellart L, Materzynska J, et al. The synthia dataset: A large collection of synthetic images for semantic segmentation of urban scenes[C]//Proceedings of the IEEE conference on computer vision and pattern recognition. 2016: 3234-3243.
[2] Richter S R, Vineet V, Roth S, et al. Playing for data: Ground truth from computer games[C]//European conference on computer vision. Springer, Cham, 2016: 102-118.
[3] Richter S R, Hayder Z, Koltun V. Playing for benchmarks[C]//Proceedings of the IEEE International Conference on Computer Vision. 2017: 2213-2222.
[4] Neuhold G, Ollmann T, Rota Bulo S, et al. The mapillary vistas dataset for semantic understanding of street scenes[C]//Proceedings of the IEEE international conference on computer vision. 2017: 4990-4999.
[5] Sakaridis C, Dai D, Van Gool L. Semantic foggy scene understanding with synthetic data[J]. International Journal of Computer Vision, 2018, 126(9): 973-992.
[6] Sakaridis C, Dai D, Hecker S, et al. Model adaptation with synthetic and real data for semantic dense foggy scene understanding[C]//Proceedings of the European Conference on Computer Vision (ECCV). 2018: 687-704.
[7] Halder S S, Lalonde J F, Charette R. Physics-based rendering for improving robustness to rain[C]//Proceedings of the IEEE/CVF International Conference on Computer Vision. 2019: 10203-10212.
[8] Yu F, Chen H, Wang X, et al. Bdd100k: A diverse driving dataset for heterogeneous multitask learning[C]//Proceedings of the IEEE/CVF conference on computer vision and pattern recognition. 2020: 2636-2645.
[9] Huang X, Wang P, Cheng X, et al. The apolloscape open dataset for autonomous driving and its application[J]. IEEE transactions on pattern analysis and machine intelligence, 2019, 42(10): 2702-2719.
[10] Sakaridis C, Dai D, Van Gool L. ACDC: The adverse conditions dataset with correspondences for semantic driving scene understanding[C]//Proceedings of the IEEE/CVF International Conference on Computer Vision. 2021: 10765-10775.
[11] Diaz-Ruiz C A, Xia Y, You Y, et al. Ithaca365: Dataset and Driving Perception Under Repeated and Challenging Weather Conditions[C]//Proceedings of the IEEE/CVF Conference on Computer Vision and Pattern Recognition. 2022: 21383-21392.
[12] Sun T, Segu M, Postels J, et al. SHIFT: A Synthetic Driving Dataset for Continuous Multi-Task Domain Adaptation[C]//Proceedings of the IEEE/CVF Conference on Computer Vision and Pattern Recognition. 2022: 21371-21382. |
|